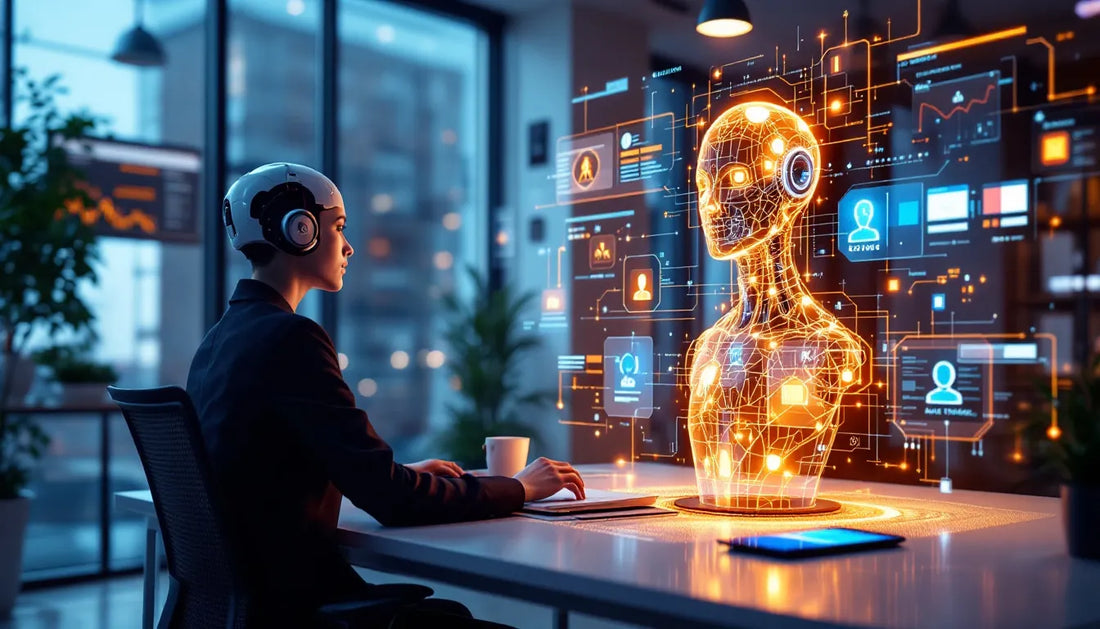
AI Agents in Finance: The Next Frontier in Algorithmic Trading
Share
As financial markets become increasingly complex, traditional algorithmic trading is evolving. The rise of AI agents — autonomous, self-improving systems — marks a new chapter in how institutions analyze, predict, and execute trades. These agents go beyond static algorithms, learning in real time, adapting to new market conditions, and collaborating across environments to maximize returns.
What Are AI Agents?
AI agents are autonomous software entities that perceive their environment, make decisions, and act to achieve specific goals. In finance, this means interpreting massive datasets, understanding economic indicators, news sentiment, and trading signals — then making trades with minimal human intervention.
Unlike traditional trading bots, AI agents can adjust their strategies over time using techniques like reinforcement learning, generative models, and memory-based decision systems. They do not follow fixed rules; instead, they learn from outcomes and optimize continuously.
Reinforcement Learning Meets Financial Markets
Firms like DeepMind, J.P. Morgan, and Citadel are exploring reinforcement learning (RL) to build AI agents capable of learning optimal policies through trial and error. An RL-based agent receives rewards or penalties based on its trading performance, gradually learning strategies that outperform benchmarks like the S&P 500.
For example, Morgan Stanley has experimented with RL models that learn to hedge portfolios dynamically based on volatility regimes and macroeconomic inputs. These agents are capable of switching strategies in real time — something traditional quant models struggle to do without explicit reprogramming.
Multimodal Decision-Making with LLMs
Large Language Models (LLMs) are now being paired with trading agents to improve reasoning over unstructured data like earnings calls, news reports, and analyst commentary. Imagine an AI agent that not only watches tick-by-tick market data but also understands when a CEO hints at margin pressure during a call and adjusts risk exposure accordingly.
BloombergGPT and other domain-specific LLMs are being integrated into financial pipelines, helping agents act with broader market context. This enables agents to make more informed decisions, especially in complex event-driven scenarios like M&A announcements or central bank interventions.
Agentic Collaboration and Portfolio Management
Advanced financial systems are experimenting with "swarms" of AI agents, each specialized in a different asset class or trading style — momentum, arbitrage, macro. These agents collaborate, share signals, and compete for capital allocation based on performance metrics. It’s a decentralized, self-managing fund driven by machines.
Funds like Man AHL and Two Sigma are reportedly testing frameworks where multiple AI agents interact across simulated environments, co-developing strategies before live deployment. This setup allows agents to stress test ideas and handle market shocks more robustly.
Regulatory and Risk Considerations
As AI agents gain autonomy, the financial industry must address new challenges. Regulators are beginning to examine how these agents make decisions, explain their logic, and avoid destabilizing effects like flash crashes. Explainability, compliance logging, and kill switches are now mandatory in most high-frequency trading environments.
Firms are also building risk firewalls that monitor AI agents in real time, pausing activity when agents diverge from expected behavior. Trust in these systems hinges on their ability to remain transparent and auditable — even when they operate at millisecond speeds across global markets.
Looking Ahead
AI agents represent the next frontier in algorithmic trading. By combining real-time learning, natural language understanding, and collaborative intelligence, they are pushing beyond the capabilities of human traders and static models. While still in early stages, the future points toward a financial ecosystem where autonomous agents continuously adapt, compete, and evolve — shaping market behavior in ways we’re only beginning to understand.