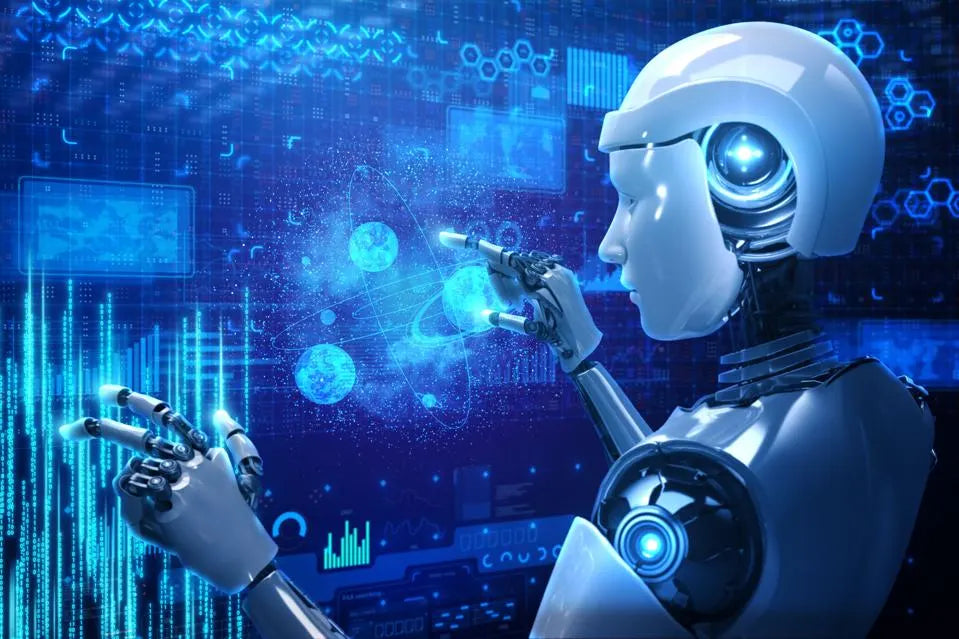
AI vs Black Swans: Can Algorithms Predict the Unpredictable?
Share
In the world of finance, a "black swan" refers to a rare and unpredictable event that has severe consequences—think the 2008 financial crisis or the COVID-19 market collapse. Traditional models often fail to detect these anomalies in time. But as artificial intelligence (AI) continues to evolve, a new question emerges: Can AI identify early signals of black swan events before they wreak havoc?
The Limits of Historical Data
Most financial models are built on historical data. However, black swans, by nature, have no precedent. Algorithms that rely solely on past market behavior are often blind to sudden shifts. This is where AI shows promise—not by predicting specific black swans, but by detecting abnormal patterns and data behaviors before human analysts can.
AI and Weak Signal Detection
Machine learning models, particularly anomaly detection systems and unsupervised learning techniques, can pick up on subtle signals in massive datasets. These "weak signals" could include unusual correlations, liquidity changes, or supply chain anomalies that precede major market events.
Example: In early 2020, some AI systems flagged supply chain disruptions in Wuhan, China, weeks before markets reacted to the COVID-19 pandemic. While they didn't predict a global crisis, the early warnings showed how machines can identify outliers missed by traditional models.
Simulating the Impossible
Reinforcement learning (RL) and generative adversarial networks (GANs) are now being used to simulate extreme market scenarios that humans might not consider. These simulations allow financial institutions to test the resilience of their portfolios and risk frameworks against a wider variety of outcomes.
Example: Hedge funds like Two Sigma and Citadel are increasingly using adversarial AI to simulate market crashes, sudden interest rate hikes, or even geopolitical events—training models to recognize "patternless" threats.
Limitations of Predictive AI
Despite these advances, it's critical to acknowledge that AI is not a crystal ball. Even the most advanced neural networks cannot predict the exact timing or nature of a black swan event. Overfitting, biased data, and model hallucinations can lead to false alarms—or worse, a false sense of security.
Human + Machine: A Hybrid Approach
The most effective strategy may not be replacing human intuition, but augmenting it. By combining AI’s ability to process vast data in real time with human judgment and scenario planning, investors can improve their readiness for unpredictable events.
The Future of Black Swan Detection
As AI tools mature, they may not prevent black swans—but they can make markets more adaptive and resilient. By spotting early warning signs, simulating edge-case scenarios, and continuously learning from new data, AI gives us a fighting chance against the unpredictable.
In an age where uncertainty is the only constant, AI's role in risk management is no longer optional—it's essential.