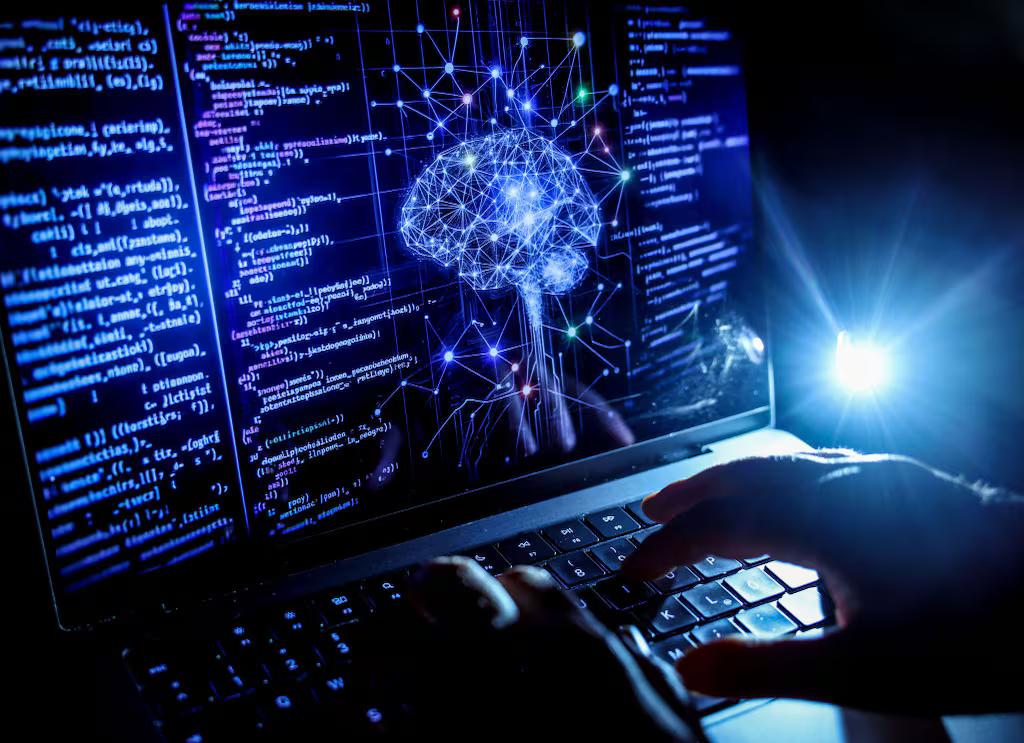
Beyond Speed: How Artificial Intelligence is Reshaping High-Frequency Trading
Share
High-frequency trading (HFT) has long been defined by speed—traders seeking to capitalize on millisecond advantages to execute countless orders per second. But the evolution of trading strategies is now being shaped by a more powerful force: artificial intelligence (AI). AI is not only enhancing the speed of trades but also transforming how trading strategies are developed, executed, and optimized. By integrating AI with HFT, traders are gaining insights into vast amounts of market data, predicting market movements more accurately, and adapting to dynamic market conditions in real-time.
The Evolution of High-Frequency Trading
High-frequency trading began with the rise of computerized algorithms designed to exploit market inefficiencies. Traders use algorithms to execute thousands of orders within fractions of a second, typically based on predefined rules that take advantage of tiny price discrepancies. These algorithms aim to make profits by operating at speeds beyond the capability of human traders.
However, as technology evolved and competition in the financial markets intensified, the need for more sophisticated and adaptive strategies became apparent. This is where AI comes in—bringing a new dimension to the speed-focused world of HFT.
The Role of Artificial Intelligence in HFT
Artificial intelligence, particularly machine learning (ML) and deep learning, is playing an increasingly important role in reshaping high-frequency trading. Here are several key ways in which AI is influencing the HFT landscape:
1. Predictive Analytics for Market Forecasting
One of the primary applications of AI in HFT is its ability to predict market movements. Traditional HFT relies on pre-programmed algorithms that react to market data in real time, but these systems can struggle to anticipate long-term market trends or sudden changes in market conditions. AI, on the other hand, can analyze large datasets—ranging from historical prices and volume patterns to news sentiment and macroeconomic indicators—and make predictions about future market movements.
Machine learning models, such as supervised learning algorithms, are trained on past data to identify patterns and make more accurate forecasts. AI’s ability to predict price movements can give traders a competitive edge, allowing them to position themselves ahead of market shifts and potentially profit from trends before they fully materialize.
2. Adaptive and Self-Learning Trading Algorithms
Unlike traditional HFT algorithms, which follow rigid rules, AI-powered trading systems are adaptive and capable of learning from their experiences. Using reinforcement learning techniques, AI systems can improve their decision-making process by learning from past actions and adjusting their strategies over time to optimize profits.
For example, a trading algorithm powered by AI can continuously assess the market’s changing conditions and adapt its approach based on the success or failure of previous trades. This self-learning capability allows the AI system to optimize trading strategies on the fly, even in volatile market conditions where traditional rules-based algorithms might struggle.
3. Sentiment Analysis and Natural Language Processing (NLP)
Another exciting aspect of AI in HFT is the integration of natural language processing (NLP), which allows machines to analyze and interpret human language. By analyzing news articles, social media posts, and financial reports in real time, AI can gain insights into market sentiment and public perception, which are often powerful indicators of market movement.
For example, a sudden change in sentiment, such as a negative news story about a particular company or sector, can cause stock prices to plummet. AI algorithms can quickly analyze this shift in sentiment and execute trades to profit from the anticipated market reaction. NLP enables AI systems to incorporate qualitative data into their decision-making process, going beyond purely quantitative market data.
4. Risk Management and Anomaly Detection
In HFT, risk management is critical. AI is helping firms detect unusual market conditions and potential risks by analyzing vast amounts of data in real time. Using anomaly detection algorithms, AI can spot patterns that deviate from normal trading behavior, alerting traders to potential issues before they become significant problems.
For instance, if a trading system detects unusual price movements or volume spikes that do not align with typical market behavior, it can automatically flag these anomalies as potential risks. AI-powered risk management tools can also monitor multiple markets simultaneously, allowing for more comprehensive risk mitigation across a trader’s portfolio.
Examples of AI in Action in High-Frequency Trading
Several leading financial institutions and trading firms are already leveraging AI to enhance their HFT strategies. Below are some examples of how AI is being applied:
- Two Sigma: Two Sigma, a quantitative trading firm, uses machine learning and AI techniques to process vast datasets and identify trading opportunities in real time. Their approach combines advanced data science with algorithmic trading to optimize strategies and improve performance.
- Citadel Securities: Citadel Securities utilizes AI to enhance its high-frequency trading strategies, including predictive analytics and risk management. Their use of AI allows them to react more quickly to changing market conditions, staying ahead of competitors in a fast-paced environment.
- DE Shaw: The DE Shaw Group, another major player in quantitative trading, applies machine learning to optimize its trading models and improve algorithm performance. The firm’s AI-driven approach helps them better understand market dynamics and adjust trading strategies accordingly.
Challenges and Considerations
While AI has the potential to revolutionize high-frequency trading, there are several challenges and considerations that need to be addressed:
- Data Quality: AI algorithms rely on vast amounts of high-quality data to make accurate predictions. Poor data quality can lead to inaccurate predictions and suboptimal trading decisions, which could result in financial losses.
- Market Regulation: The introduction of AI in HFT raises concerns about market fairness and transparency. Regulators are scrutinizing the impact of AI-driven trading strategies on market stability, particularly as these algorithms become more complex and autonomous.
- Computational Complexity: AI algorithms, especially deep learning models, can be computationally intensive, requiring significant processing power and specialized hardware. Financial firms need to ensure they have the infrastructure to support AI models in real-time trading environments.
Conclusion: The Future of AI in High-Frequency Trading
Artificial intelligence is fundamentally changing the landscape of high-frequency trading. By moving beyond speed and embracing advanced predictive analytics, self-learning algorithms, and natural language processing, AI is enabling traders to execute smarter, more adaptive trading strategies. While there are challenges to overcome, the potential for AI to optimize trading performance, manage risk, and create new opportunities in the financial markets is vast. As AI continues to evolve, we can expect to see even more transformative changes in the way high-frequency trading operates, reshaping the future of financial markets.